Materials Design and Informatics Group
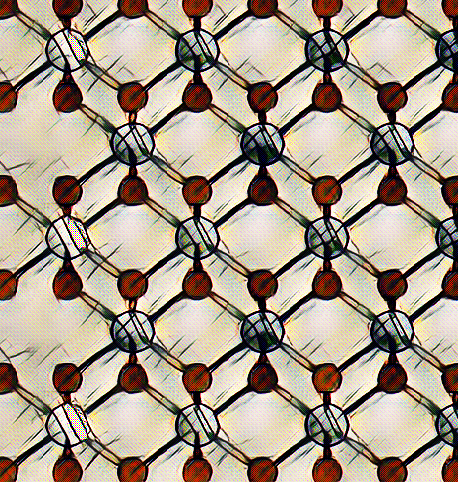
Department of Chemistry
University College London
London WC1H 0AJ
Materials Design and Informatics Group (MDIG) is a research collective, working to accelerate develoment of new green energy materials. We are based at UCL, in the Department of Chemistry.
We use a combination of data-driven methods (such as deep learning and Bayesian statistics) and quantum mechanics calculations to design new materials on computers and to help accelerate the experimental characterisation of materials. We work with other academics, national facilities and companies.
news
Apr 3, 2025 | New paper alert - REMatch plus SOS: Machine-learning-accelerated structure prediction for supported metal nanoclusters |
---|---|
Jan 27, 2025 | New paper alert - On the use of clustering workflows for automated microstructure segmentation of analytical STEM datasets |
Jan 20, 2025 | Welcoming Ahmed Ismail who is joining us as a Daphne Jackson Research Fellow. |
Oct 1, 2024 | Welcoming Cyprien Bone, who is joining us as a PhD student and Bradley Martin who is joining as a Research Fellow. |
Sep 12, 2024 | New paper alert - Predicting Colloidal Interaction Parameters from Small-Angle X-ray Scattering Curves Using Artificial Neural Networks and Markov Chain Monte Carlo Sampling |
selected publications
- Computational screening of all stoichiometric inorganic materialsChem 2016
- Machine learning for molecular and materials scienceNature 2018
- Designing interfaces in energy materials applications with first-principles calculationsnpj Computational Materials 2019
- Distributed representations of atoms and materials for machine learningnpj Computational Materials 2022
- Entropy-based active learning of graph neural network surrogate models for materials propertiesThe Journal of Chemical Physics 2021
- Interpretable and explainable machine learning for materials science and chemistryAccounts of Materials Research 2022